AI: Saving Grace or Sworn Enemy of the Environment?
- shiradickler9
- Nov 19, 2024
- 6 min read
Updated: Nov 25, 2024
Balancing AI’s Role in Tackling Climate Risks While Managing Its Environmental Footprint
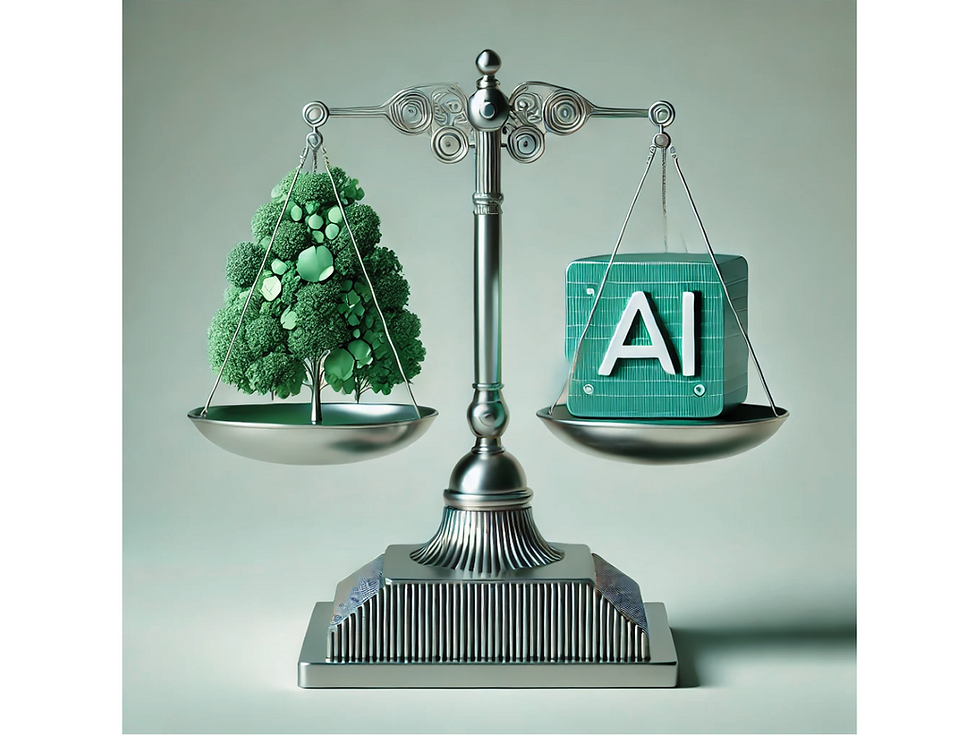
TL;DR
AI has a dual impact on sustainability: while its energy-intensive processes contribute to significant environmental costs, it also holds immense potential to help businesses anticipate climate risks, reduce supply chain emissions, and build long-term resilience.
By adopting responsible practices—like transparent energy use reporting and leveraging platforms like ECO-OS for data-driven ESG management—companies can harness AI’s strengths to drive sustainable growth while mitigating its drawbacks. The key lies in balancing innovation with environmental responsibility.
In the past year, the headlines have buzzed with doom-filled predictions about the environmental cost of artificial intelligence (AI). From skyrocketing energy demands to massive water consumption, the narrative paints AI as a ravenous beast consuming the planet's resources at an alarming rate. For businesses who stand to gain from incorporating AI into their operations, this raises an important question: does AI support or hinder sustainability goals?
The answer is complex. Yes, AI demands significant resources, but it also holds vast potential to enhance sustainability efforts, especially in manufacturing. As we explore this dual nature, let’s consider how AI, when used wisely, can shift from a resource burden to a powerful ally in achieving sustainable business practices.
The Environmental Impact of AI
AI, especially machine learning, is energy-hungry. Teaching an AI to think is akin to raising a child. It requires feeding vast amounts of data to models that need countless transactions to "learn" effectively. Training a single AI model can produce as much carbon as five cars over their entire lifetimes. And generative AI—a rapidly growing sector—can use up to 30 times more energy than conventional computing tasks. This resource demand is amplified by the infrastructure needed to support AI, including vast data centers and server farms that require land, electricity, and cooling systems.
In many ways, this environmental footprint mirrors that of manufacturing itself. Just as manufacturing has evolved to address its carbon emissions and energy demands, the AI sector must now address its own sustainability challenges.
The Sustainability Challenge: High Energy Costs and Carbon Emissions
With such a high environmental cost of AI, it seems like rapid growth in this technology might not coexist with sustainability goals. Like many technologies in their infancy, AI’s development has prioritized speed and capability over sustainability, and the climate burden it carries could grow unchecked unless we act now.
(As we wrestle with these questions, it's important to step back and reflect on the broader landscape. The environmental concern surrounding AI is not new—it’s part of a larger story that began long before AI became a buzzword. The environmental footprint of digital technologies has been a growing issue for decades, sparked by the exponential rise of cloud computing, machine learning, and blockchain and cryptocurrencies. AI, with its high energy, land, and water requirements to maintain vast server farms, only intensifies the conversation.)
Some leaders, like OpenAI CEO Sam Altman, propose bold solutions such as nuclear fusion to power AI. But realistically, the more immediate solution lies in adopting the same practices used in energy-intensive industries: transparent reporting of energy and water use, prioritizing energy-efficient technology, and using renewable energy sources. This approach is familiar to manufacturing leaders, who have implemented similar practices over the past decade to reduce their environmental impact. By extending these principles to the AI industry, we can harness AI’s benefits without allowing its environmental costs to grow unchecked.
Harnessing AI for Sustainability in Manufacturing
Although AI’s energy demands are high, the technology offers powerful tools to solve complex sustainability challenges, especially for manufacturers. Earth’s ecosystems are vast, interconnected, and incredibly complex—making them difficult for humans to fully understand, let alone protect. AI, with its unparalleled ability to process and analyze massive datasets, can help us model, predict, and mitigate environmental risks in ways that were previously unimaginable.
For example, machine learning models have helped predict and manage large-scale events like disease outbreaks and environmental hazards. In one landmark study, AI was used to predict a cholera outbreak in Colombia three years in advance by analyzing data on weather patterns and governance issues. Similarly, manufacturing companies can use AI to model and predict risks in their supply chains, operations, and environmental footprint, positioning themselves to respond proactively to sustainability challenges.
AI's Role in Mitigating Second-Order Environmental Risks
The power of AI in driving sustainability lies not only in addressing immediate, or “first-order,” risks, such as direct greenhouse gas emissions but also in understanding complex, indirect, or “second-order,” risks. First-order risks are direct outcomes of events, while second-order risks are long-term impacts that may be less obvious but are crucial for strategic planning.
Consider the impact of rising sea levels. While coastal flooding is an immediate concern, secondary effects—like community displacement, infrastructure loss, and saltwater intrusion into freshwater sources—create further challenges for manufacturers reliant on stable infrastructure and consistent water supplies. AI enables companies to analyze vast amounts of data, identifying these second-order risks and their potential effects on long-term operations. With AI, manufacturers can look beyond immediate threats to make informed, proactive decisions that protect both business continuity and environmental resilience.
ECO-OS: Turning Data into Sustainability Action
To achieve tangible sustainability goals, businesses need solutions that translate insights into actionable steps. This is where ECO-OS provides value. By combining machine learning with detailed operational data, ECO-OS enables companies to assess second- and third-order environmental risks specific to their operations.
On the ECO-OS platform, for instance, companies can input data about individual sites, machinery, and production lines from all over the world to receive tailored insights into environmental risks and compliance requirements, centralize Scope 3 emissions data, model decarbonization scenarios and generate predictions to determine if they are on track to meet their ESG goals.
For sustainability-minded businesses, this approach means smarter, data-driven decisions that directly support ESG goals, avoiding greenwashing and staying ahead of regulatory pressures (like the new Corporate Sustainability Reporting Directive) by mapping out clear action plans based on real-time, quantitative data, and driving real business value.
Regulating AI for Long-Term Impact
Fostering a symbiotic relationship between AI and sustainability isn’t solely about waiting for groundbreaking energy solutions. More immediately, it’s about adopting responsible practices—transparent energy and water reporting, prioritizing efficient hardware and algorithms, and using renewable energy—to support AI’s growth in a way that aligns with sustainability standards.
As we have seen in the world of manufacturing, responsible management of environmental impacts is not only possible but essential for long-term resilience. Applying these principles to the AI sector can ensure that its growth does not contribute further to climate challenges but instead supports a sustainable path forward.
Final Thoughts: AI—Friend or Foe for the Environment?
So, is AI a friend or foe to the climate? Like most powerful tools, the answer depends on how we use it. The environmental cost of AI is undeniable, but its potential to drive sustainability in manufacturing is equally promising. As we innovate, the challenge will be to harness AI’s strengths—like the actionable insights provided by ECO-OS platform—while mitigating its weaknesses. In doing so, industry leaders can make this technology a cornerstone of a more sustainable, resilient future.

Glossary
ESG (Environmental, Social, and Governance): A comprehensive framework used to evaluate a company's commitment to sustainable and ethical practices. ESG encompasses three key areas: Environmental factors, such as a company's carbon footprint, resource usage, and environmental stewardship; Social factors, including employee relations, community involvement, and human rights; and Governance factors, which assess corporate leadership, transparency, ethical behavior, and stakeholder engagement. ESG criteria help stakeholders understand a company's long-term sustainability and ethical impact on the world, guiding investment and operational decisions.
Greenhouse gasses (GHGs): Gasses that allow light and heat from the sun to enter the earth's atmosphere, but trap heat on earth, preventing it from being released back into space. These gasses are believed responsible for human-caused (anthropogenic) climate change. The main GHGs that have an effect on climate change include: carbon dioxide (CO2), nitrous oxide (N2O), methane gas (CH4), refrigerant gasses (HFCs).
Scope 3 emissions: Indirect emissions involved in producing a product, including upstream and downstream emissions. Upstream emissions relate to those activities that support the production of your product before manufacturing (i.e. production and transportation of raw materials, business travel, capital goods, etc.) and Downstream emissions support the distribution and end-of-life of your product (processing of sold products, waste disposal, use of product, etc.) Scope 3 emissions are often the hardest to collect and typically account for the majority of emissions stemming from a product's production (for non-vertically integrated companies.
Greenwashing: A phenomenon where a company makes misleading or false claims to convince consumers that its products are more environmentally friendly or have a greater positive environmental impact than they truly do. This is often viewed as a critique of Environmental, Social, and Governance (ESG) practices because it undermines the credibility and effectiveness of ESG efforts.
Corporate Sustainability Reporting Directive (CSRD): An obligatory reporting framework enacted by the European Parliament that applies to certain companies with operations taking place in the European Union. The mandate requires companies to report on specific ESG metrics, conduct a double materiality assessment (considering both the impact of the company on the environment and the impact of environmental issues on the company), and undergo third party assurance to verify the accuracy and reliability of their ESG disclosures.
Double Materiality Assessment: Evaluates not just how the company’s operations affect the broader environment and society, but how external environmental and social issues may impact the company's financial performance and reputation. The CSRD mandate requires companies to report only on ESG issues deemed material, supported by their double materiality assessments. This approach provides a comprehensive understanding of risks and opportunities essential for navigating today's business landscape.
Kommentare